Apache Kafka : Distributed Messaging System
Apache Kafka is a distributed publish-subscribe messaging system which can handle a large volume of data and can send data from one point to another point. Kafka messages are persisted on the disk and replicated within the cluster to prevent data loss Kafka is a fast, scalable, distributed in nature by its design, partitioned and replicated commit log service.
Reliability : Kafka is distributed, partitioned, replicated and fault tolerance.
Scalability : Kafka messaging system scales easily without down time..
Durability : Kafka uses Distributed commit log which means messages persists on disk as fast as possible, hence it is durable..
Performance : Kafka has high throughput for both publishing and subscribing messages. It maintains stable performance even many TB of messages are stored.
Benefits of using Kafka :
Scalability : Kafka messaging system scales easily without down time..
Durability : Kafka uses Distributed commit log which means messages persists on disk as fast as possible, hence it is durable..
Performance : Kafka has high throughput for both publishing and subscribing messages. It maintains stable performance even many TB of messages are stored.
In comparison to other messaging systems, Kafka has better throughput, built-in partitioning, replication and inherent fault-tolerance, which makes it a good fit for large-scale message processing applications.
Kafka Architecture
Before understanding the architecture of Kafka , we must be clear with few components used in Kafka.
Message : A message is a payload of bytes saved in Topic.
Topic : A stream of message of a particular category is called Topic. Producer publish the message to the topic.
Producer : A Producer can be anyone who can publish messages to a Topic.
Consumer : A Consumer can subscribe to one or more Topics and consume the published Messages by pulling data from the Brokers.
Kafka Cluster : Kafka cluster is a set of server , each of which is called Broker.
ZooKeeper : ZooKeeper is used for managing and coordinating Kafka broker. ZooKeeper service is mainly used to notify producer and consumer about the presence of any new broker in the Kafka system or failure of the broker in the Kafka system. As per the notification received by the Zookeeper regarding presence or failure of the broker then producer and consumer takes decision and starts coordinating their task with some other broker.
Producers : Producers push data to brokers. When the new broker is started, all the producers search it and automatically sends a message to that new broker. Kafka producer doesn’t wait for acknowledgements from the broker and sends messages as fast as the broker can handle.
Consumers : Since Kafka brokers are stateless, which means that the consumer has to maintain how many messages have been consumed by using partition offset. If the consumer acknowledges a particular message offset, it implies that the consumer has consumed all prior messages. The consumer issues an asynchronous pull request to the broker to have a buffer of bytes ready to consume. The consumers can rewind or skip to any point in a partition simply by supplying an offset value. Consumer offset value is notified by ZooKeeper.
Kafka Storage
Kafka is distributed in nature, a Kafka cluster typically consists of multiple brokers. To balance load, a topic is divided into multiple partitions and each broker stores one or more of those partitions. Multiple producers and consumers can publish and retrieve messages at the same time. See the below image for partitioning and replication of data.
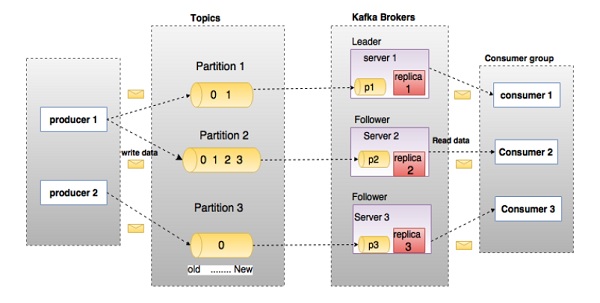
In the above architecture , kafka cluster has 3 brokers and a topic has been created with 1 partition and replication factor 3 which means that if the replication factor of the topic is set to 3, then Kafka will create 3 identical replicas of each partition and place them in the cluster to make available for all its operations. To balance a load in cluster, each broker stores one or more of those partitions. Multiple producers and consumers can publish and retrieve messages at the same time.
Let's take another example to make it more clear suppose if our kafka cluster has 4 broker and we create a topic with 2 partition and replication factor 4. Then for that particular topic 2 partition will be created with 4 replica of each partition. Which means total 4*2 =8 partition will be created. To balance the load 4 replica for partition-0 will be created on different 4 broker and same for the partition-1.
There are few rules which zookeeper follows during partitioning of a topic.
- Assume, if there are N partitions in a topic and N number of brokers, each broker will have one partition.
- Assume if there are N partitions in a topic and more than N brokers (n + m), the first N broker will have one partition and the next M broker will not have any partition for that particular topic.
- Assume if there are N partitions in a topic and less than N brokers (n-m), each broker will have one or more partition sharing among them. This scenario is not recommended due to unequal load distri-bution among the broker.
Retention policy :
Retention policy governs how Kafka retains messages.You specify how much data or how long data should be retained, after which Kafka purges messages in-order—regardless of whether the message has been consumed.
Partitions are split into segments
Partitions are split into segmentsKafka needs to regularly find the messages on disk that need purged. With a single very long file of a partition’s messages, this operation is slow and error prone. To fix that (and other problems we’ll see), the partition is split into segments.
When Kafka writes to a partition, it writes to a segment — the active segment. If the segment’s size limit is reached, a new segment is opened and that becomes the new active segment.
Segments are named by their base offset. The base offset of a segment is an offset greater than offsets in previous segments and less than or equal to offsets in that segment.
On disk, a partition is a directory and each segment is an index file and a log file.
$ tree kafka | head -n 6 kafka ├── events-1 │ ├── 00000000003264504070.index │ ├── 00000000003264504070.log │ ├── 00000000003265011412.index │ ├── 00000000003265011412.log
Segment logs are where messages are stored
Each message is its value, offset, timestamp, key, message size, compression codec, checksum, and version of the message format.
The data format on disk is exactly the same as what the broker receives from the producer over the network and sends to its consumers. This allows Kafka to efficiently transfer data with zero copy.
$ bin/kafka-run-class.sh kafka.tools.DumpLogSegments --deep-iteration --print-data-log --files /data/kafka/events-1/00000000003065011416.log | head -n 4 Dumping /data/kafka/appusers-1/00000000003065011416.log Starting offset: 3065011416 offset: 3065011416 position: 0 isvalid: true payloadsize: 2820 magic: 1 compresscodec: NoCompressionCodec crc: 811055132 payload: {"name": "Travis", msg: "Hey, what's up?"} offset: 3065011417 position: 1779 isvalid: true payloadsize: 2244 magic: 1 compresscodec: NoCompressionCodec crc: 151590202 payload: {"name": "Wale", msg: "Starving."}
Segment indexes map message offsets to their position in the log
The segment index maps offsets to their message’s position in the segment log.
The index file is memory mapped, and the offset look up uses binary search to find the nearest offset less than or equal to the target offset.
MessageId in kafka
Unlike traditional message system, a message stored in Kafka system doesn’t have explicit message ids.Messages are exposed by the logical offset in the log. This avoids the overhead of maintaining auxiliary, seek-intensive random-access index structures that map the message ids to the actual message locations. Messages ids are incremental but not consecutive. To compute the id of next message adds a length of the current message to its logical offset.
How consumer consume message in kafka
Consumer always consumes messages from a particular partition sequentially and if the consumer acknowledges particular message offset, it implies that the consumer has consumed all prior messages. Consumer issues asynchronous pull request to the broker to have a buffer of bytes ready to consume
Comments
Post a Comment